Fireworks: Production Deployments for the Compound AI Future
The advent of intelligence as an API ushered in an explosion in AI—and Fireworks is emerging as a leader in the market.
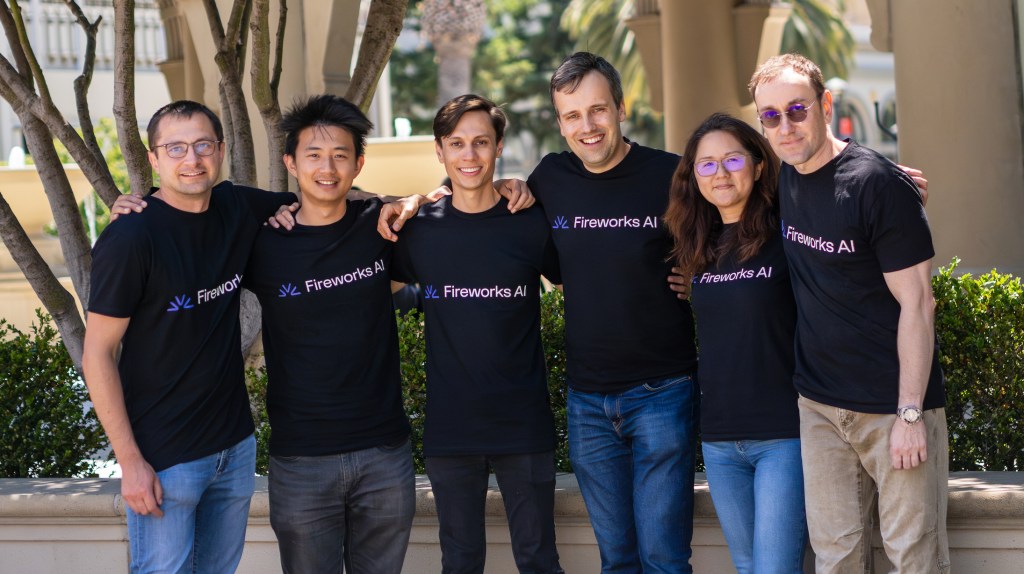
It is a similar arc at every company: developers begin their AI journey using a “Cadillac”—a very large, closed-source model to bootstrap a demo. But as they prototype to product-market fit and start to move their AI prototypes into production, they quickly start stumbling over a common set of hurdles:
- Latency: Customers expect applications close to real time, and milliseconds can degrade their experience—and their conversion.
- Cost: At production volume and scale, closed-source model bills rack up quickly.
- Quality: For some tests, a larger model may underperform relative to a smaller, finely tuned model with specific examples.
- Model and data ownership: Many enterprises are wary of sharing their secret sauce with an external provider, and prefer to host inference within their own virtual private cloud.
- External dependencies: Companies want multiple models from a reliable cloud service provider, with no single source of failure for critical applications.
In short, once companies hone in on a specific AI use case, the “Cadillac” often becomes overkill: too heavy, too slow and too expensive to best serve production workloads.
Meanwhile, teams are also increasingly shifting to compound AI systems, which combine multiple model calls, retrievers or external tools, offer better performance, reliability and control, versus relying on a single model.
A growing need
Thus enterprises are balancing a constellation of needs as they enter production: they crave the ease of a closed-source model provider; the latency, cost, quality and security that open-source models offer; and the versatility of compound systems. We at Sequoia have noticed a sharp inflection point over the last 6-9 months as more open-source alternatives have become viable.
It is a massive opportunity. The AI compute market alone is measured in tens of billions of dollars annually, and we expect a shift from majority training to majority production over the next several years. We also believe that the tailwinds behind the AI inference market are as durable as they are large. Silicon Valley has a long history of infrastructure providers making ever-more-ambitious applications possible by pushing the price-performance curve. By meeting the pragmatic needs of enterprises and pushing the boundaries of that curve, Fireworks has a chance to become a defining infrastructure provider for the age of AI.
An emerging leader
This market is not only large, but brutally competitive, with every startup claiming to have the best latency, throughput and cost. But as we talked with AI companies in our portfolio, a crowded market started to become crystal clear: Fireworks was the emerging market leader, with the best technology and the best team.
Co-founders Benny Chen, Chenyu Zhao, Dmytro Dzhulgakov, Dmytro Ivchenko, James Reed, Lin Qiao and Pawel Garbacki bring together deep expertise from Google and Meta, including leadership of Meta’s PyTorch, which became the dominant framework for training neural nets including all modern large language models and diffusion models. Now, the Fireworks team is using those learnings to build a proprietary inference stack. And just as PyTorch was known for, among other things, its performance, which required multiple layers of optimization between hardware and framework, Fireworks’ custom CUDA kernel FireAttention has exceptional performance compared to other providers’ open source inference and serving frameworks. In particular, FireAttention v2 is the leading low-latency inference engine for real-time applications, with speed improvements up to 8x and beyond.
What’s more, their latest offering is purpose-built to address the growing shift toward compound AI systems. FireFunction V2, an open-weight function-calling model, can orchestrate across multiple models, as well as their external data and knowledge sources and other APIs. It also seamlessly integrates with a range of tools and frameworks, allowing developers to quickly and easily build scalable multi-inference workflows.
Fireworks is also approaching this market holistically; rather than simply providing a suite of tools (e.g., inference and fine-tuning), they are building a full solution that delivers the optimal mixture of models and functions based on customers’ specific requirements. And by choosing to partner, rather than compete, with cloud providers and enabling deployment into existing virtual private clouds, they have created a win-win for customers. Cloud vendors can focus on hardware purchasing, data center capex and service availability, while Fireworks can focus on delivering the most performant inference engine and the best developer experience for Generative AI. They’ve made running popular open-source models inside your cloud provider not only faster and cheaper, but more ergonomic, as well.
Grand ambitions
Already, the customers Fireworks has signed reflect its stellar reputation. Following competitive bake-offs, enterprises (Uber, DoorDash, Upwork, Quora) and AI-native startups (Cursor, Superhuman, Sourcegraph Cody, Cresta) alike have chosen to build their stack around Fireworks. The platform is making it easier for these to achieve large model performance from small model cost and latency—and to build agentic workflows that utilize specific underlying models.
But Fireworks’ vision is not simply to provide the fastest inference engine. In addition to FireFunction V2, recent product announcements such as FireOptimus, an LLM inference optimizer that learns your traffic to provide better latency (2x) and quality (>= GPT-4), offer a glimpse of the innovations ahead.
In the months and years to come, the grand ambition is to build the single best platform enterprises can rely on as they deploy AI into production. We are excited to double-down on our partnership with Lin and the rest of the team as we lead Fireworks’ Series B, and to continue supporting the growth of both the platform and the team. As more companies make the choice that latency, cost, quality, ownership and developer experience matter for production AI—and as compound systems expand access to the sophisticated solutions once available to only a select few—the underlying technology this team is building will keep getting better, enabling an ever-expanding set of delightful customer experiences.